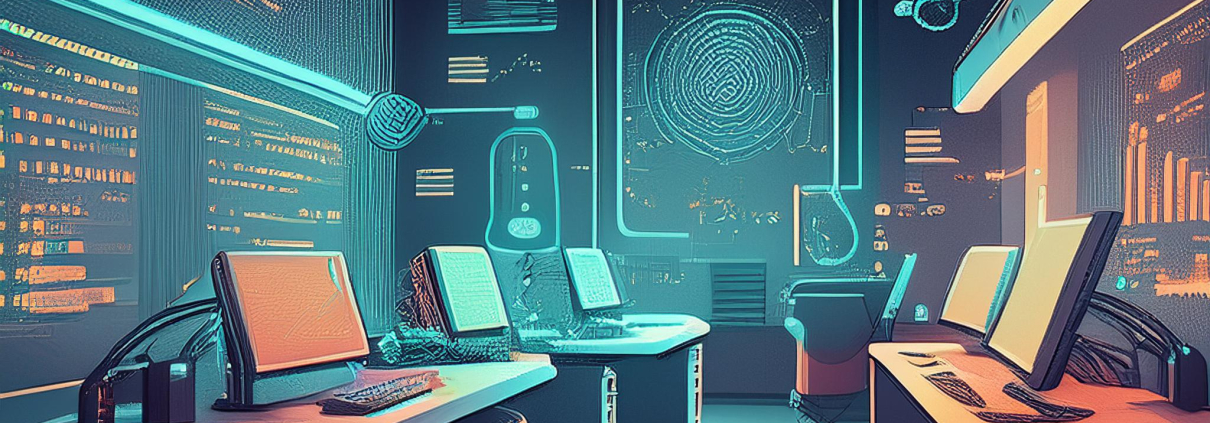
Machine Learning and Data Analytics Group (MLDAG)
Director: Ananda Mohan Mondal, Ph.D.
Research Focus:
- Artificial Intelligence and Machine Learning
- Computational Biology
- Health Informatics
Dr. Mondal’s work has been centered on raising the profile of health informatics research through the body of his work at the intersection of computational biology and artificial intelligence, with particular interest in algorithmic cancer biology, machine learning, explainable AI (XAI), and precision medicine. Cancer is a complex, multifactorial disease. Advances in computational biology and AI have pushed the frontiers of our understanding in this field at a tremendous pace. Dr. Mondal is currently focused on studying the discovery of cancer biomarkers and estimation of intratumor heterogeneity (ITH) with the long-term goal of addressing basic questions in cancer precision medicine: how does a tumor evolve? How can we study drug response? How can we address drug resistance? His research themes can be divided into two dimensions: Methodology Development based on graph theory, feature selection, XAI, and cooperative game theory; Applications to Precision Medicine, including disease biomarker discovery, estimation of the level of ITH contributing toward tumor evolution, drug resistance, and drug response. Dr. Mondal’s immediate plan is to expand the horizon of his algorithmic cancer biology research to study the impacts of climate change on the cancer control continuum. Another direction is to develop AI-based tools to analyze both omics and image data for the discovery of biomarkers for neurodegenerative diseases.
Dr. Mondal’s research themes have successfully secured four PI grants and two Co-PI grants from Florida Department of Health (FDOH), National Institute of Health (NIH), and National Science Foundation (NSF), including the prestigious NSF CAREER RUI (Research in Undergraduate Institute) award. These grant awards have brought in a substantial ~$3.37M in funding to FIU and underscore the importance and potential of his work. The funded research projects are briefly enumerated below in reverse chronological order:
Project-4: NIH R21: Explainable AI-Based Multi-Omics Analysis of Lung Cancer Health Disparity.
Major Goals: This project aims to understand and discover the biological factors of health disparities in lung cancer between African Americans and European Americans at the multi-omics level. An explainable artificial intelligence-based computational framework will be developed using a cooperative game theory-based machine learning approach, first, to identify patient-specific tumor heterogeneity or disparity information, second, to identify cohort-specific disparity information, and third, to discover disparity between two cohorts. The developed tool will help decipher the race- and sex-specific risk factors such as African American Males, European American Males, African American Females, European American Females, etc., which will guide better treatment strategy, thus, benefitting humanity.
Project-3: FDOH: Next‐Generation Biomedical Big Data Platform for Cancer Research and Collaboration Across Florida.
Major Goals: The goal of this project is to set up a Florida-wide Cancer data portal, with access to anonymized data and software analysis tools.
Project-2: NIH Pilot: Deep Learning to Discover the Disparities in Lung Cancer between African American and European American Males.
Major Goals: The goal is to develop predictive models based on generative adversarial networks (GANs) that can differentiate the transcription state alterations between cancer and normal tissues of each individual from the African American Male and European American Male cohorts. This information will allow us to decipher both common and race-specific risk factors of lung cancer development in African American Males and European American Males.
Project-1: NSF CAREER-RUI: NetDA—Protein Network-Based Software for Disease Analysis Using Cliques, Bipartite Graphs, and Diffusion Kernels.
Major Goals: The major goals of this project are to identify the protein network biomarkers and explore the disease progression at the protein network level for five cancers, including breast, colon, liver, lung, and lymphoma.